Artificial Intelligence (AI) has emerged as a transformative force in various industries, and healthcare is no exception. In recent years, AI technologies have gained traction in medical practice, offering unprecedented opportunities to improve patient care, enhance clinical decision-making, and streamline healthcare operations. However, the integration of AI in medical practice also presents challenges and limitations that must be carefully addressed. In this article, we will explore the opportunities and limitations of utilizing artificial intelligence in medical practice, highlighting its potential to revolutionize healthcare delivery while acknowledging the ethical, technical, and regulatory considerations that accompany its adoption.
Opportunities of AI in Medical Practice:
- Enhanced Diagnostic Accuracy:
One of the primary opportunities of AI in medical practice is its ability to enhance diagnostic accuracy and efficiency. AI-powered diagnostic algorithms can analyze medical imaging scans, laboratory tests, and patient data with remarkable speed and precision, aiding healthcare providers in making more accurate and timely diagnoses. By leveraging machine learning and deep learning techniques, AI algorithms can identify patterns, anomalies, and predictive biomarkers that may not be readily apparent to human observers, thereby improving diagnostic outcomes and patient care.
- Personalized Treatment Planning:
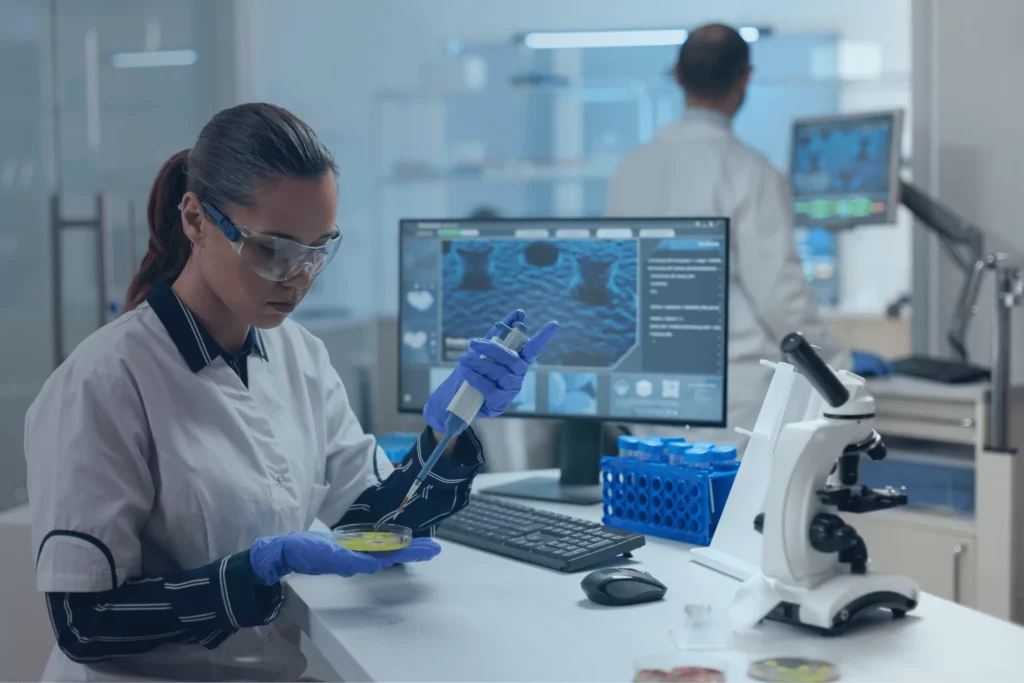
AI has the potential to revolutionize treatment planning by enabling personalized and tailored approaches to patient care. By analyzing large datasets of patient health records, genomic data, and clinical outcomes, AI algorithms can identify individualized treatment strategies based on a patient’s unique characteristics, genetic makeup, and medical history. This personalized medicine approach holds promise for optimizing therapeutic interventions, minimizing adverse reactions, and improving patient outcomes across a wide range of medical conditions.
- Predictive Analytics and Risk Stratification:
AI-powered predictive analytics and risk stratification models can help healthcare providers identify patients at high risk of developing certain diseases or adverse health outcomes. By analyzing patient data in real-time, AI algorithms can detect early warning signs, identify trends, and predict future health events, enabling proactive interventions and preventive measures. Moreover, AI-driven risk stratification tools can assist healthcare providers in triaging patients, allocating resources, and optimizing care delivery workflows to improve patient outcomes and healthcare efficiency.
- Clinical Decision Support Systems:
AI-based clinical decision support systems (CDSS) offer valuable support to healthcare providers by providing evidence-based recommendations, treatment guidelines, and decision-making tools at the point of care. CDSS leverage AI algorithms to analyze patient data, medical literature, and clinical guidelines, offering actionable insights and suggestions to guide clinical decision-making. By integrating seamlessly into electronic health record (EHR) systems and workflow processes, AI-driven CDSS can help reduce medical errors, enhance patient safety, and improve the quality of care delivery.
Limitations and Challenges of AI in Medical Practice:
- Data Quality and Interoperability:
One of the primary challenges of utilizing AI in medical practice is ensuring the quality, integrity, and interoperability of healthcare data. AI algorithms rely on large volumes of high-quality data to train and validate models effectively. However, healthcare data are often fragmented, incomplete, and heterogeneous, making it challenging to standardize and harmonize data across different sources and systems. Moreover, issues related to data privacy, security, and consent pose additional hurdles to the widespread adoption of AI in healthcare.
- Interpretability and Transparency:
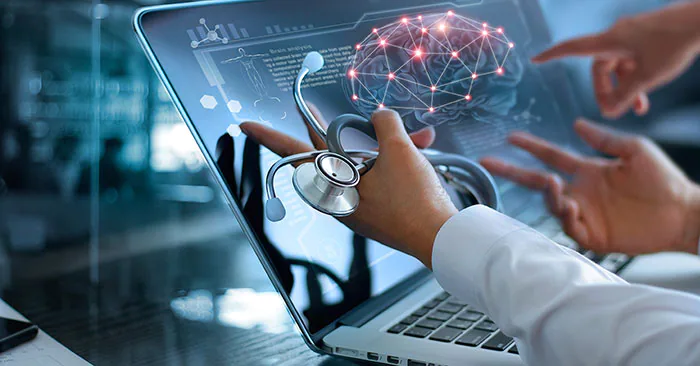
Another limitation of AI in medical practice is the lack of interpretability and transparency in AI algorithms. Deep learning models, in particular, are often regarded as “black box” systems, meaning that the rationale behind their predictions and recommendations is not readily interpretable by human users. This lack of transparency raises concerns about the accountability, trustworthiness, and ethical implications of AI-driven decision-making in healthcare. Addressing these concerns requires developing explainable AI techniques and fostering transparency in AI model development and deployment.
- Regulatory and Ethical Considerations:
AI applications in medical practice are subject to stringent regulatory oversight and ethical scrutiny to ensure patient safety, privacy, and equity. Regulatory agencies such as the U.S. Food and Drug Administration (FDA) and the European Medicines Agency (EMA) impose rigorous standards for the development, validation, and deployment of AI-based medical devices and software. Moreover, ethical considerations such as bias, fairness, and accountability must be carefully addressed to mitigate the potential risks and unintended consequences of AI in healthcare.
- Integration and Adoption Challenges:
Integrating AI technologies into existing healthcare systems and workflows poses significant challenges related to interoperability, usability, and user acceptance. Healthcare providers may encounter resistance to change, workflow disruptions, and technical barriers when implementing AI solutions in clinical practice. Moreover, the complexity and heterogeneity of healthcare environments require tailored approaches to AI integration and adoption, including comprehensive training programs, stakeholder engagement, and change management strategies.
In conclusion, artificial intelligence holds tremendous promise for transforming medical practice and improving patient care across the continuum of healthcare delivery. By harnessing the power of AI, healthcare providers can enhance diagnostic accuracy, personalize treatment planning, predict health outcomes, and optimize clinical decision-making. However, the successful integration of AI in medical practice requires addressing a myriad of challenges and limitations, including data quality, interpretability, regulatory compliance, and integration issues. Moving forward, stakeholders must work collaboratively to overcome these barriers and leverage AI technologies responsibly and ethically to advance healthcare delivery and improve patient outcomes.